The goal of this study was to take a machine learning network neuroscience approach to understanding how the brain supports learning in adolescents and young adults.
Background: Time-varying changes in patterns of functional connectivity can reflect the dynamics of task demands. By formally encoding such changes in network models and applying tools from graph theory, prior work has demonstrated that dynamic network flexibility (DNF) tracks the course of learning (Bassett et al., 2011). In adults, DNF may be a mechanism underlying the integration of information necessary for learning. Specifically, DNF in striatal-cortical circuits in adults relates to better learning and whole-brain DNF is correlated with individual differences in reinforcement learning model parameters (Gerraty et al., 2018). Adolescents have shown better learning on this same task; however, it is unknown how DNF supports reinforcement learning during adolescence. Here we compared DNF across the whole brain in adolescents (13-17 years) to adults (24-30 years) and examine DNF in learning related regions (striatum, hippocampus, prefrontal cortex, and sensory regions) as participants engaged in a reinforcement learning task. Results will inform the role of DNF in complex learning involving information integration and reward sensitivity in adolescents.
Findings and Implications:
There were no significant age group differences (between adolescents and adults) in dynamic neural network flexibility during learning
Group differences in learning did exist and are not because of greater dynamic network flexibility of the adolescents' brains
Dynamic network flexibility of sensory regions (auditory, visual, motor) differed across adolescents vs. adults while learning in an fMRI (functional magnetic resonance imaging) scanner
Supporting prior research in adults, the striatum plays a key, flexible role in information integration during learning in adolescents
Network Neuroscience Methodology (tools utilized: FSL/MATLAB):
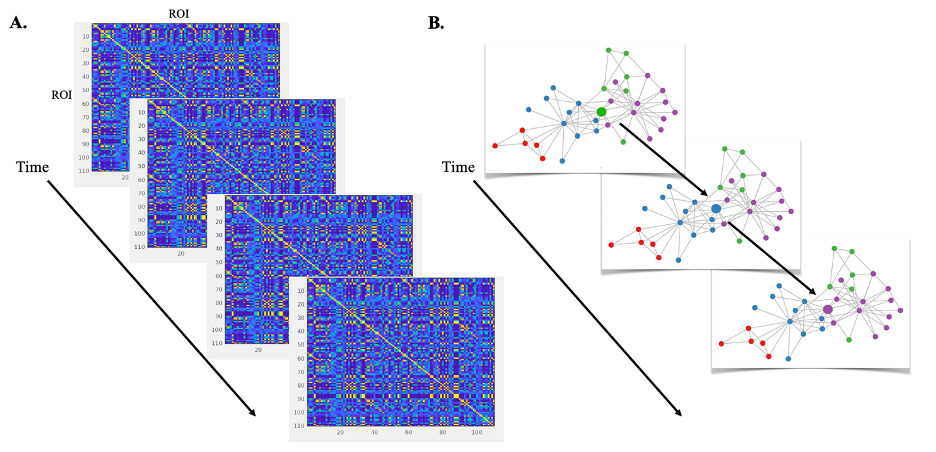
Graph theoretical neural network organization of the brain. A. Functional connectivity across all 110 ROIs. Each ROIs functional connectivity is extracted with every other ROI, represented by colored squares. B. Community detection demonstrates network organization across all ROIs. Nodes (colored dots) represent individual ROIs, and edges (gray lines) represent the functional connectivity between nodes. Modularity is the topological organization of whole human brain functional networks and the partitioning of these networks into a set of modules. Each module, or community (represented by distinct colors), is defined by dense internal or intra-modular connectivity and relatively sparse external or inter-modular connectivity (Meunier et al., 2009; Newman & Girvan, 2004). Dynamic network flexibility (DNF) is a measure of how frequently an individual node, or ROI, changes its functional connectivity with assigned modules across time windows (e.g., a., b., c.). The node in the center of each time window represents a given ROI, e.g., the striatum. DNF is demonstrated by the node in the center of the graph changing community assignment across learning (time).
Statistical Modeling Approach (using R)
Bayesian mixed-effects logistic models, using the brms package in R, were used to test the within-subject and between age group variances across learning blocks. Each repeated measures trial included an outcome binary response variable of 1 = correct response, 0 = incorrect response that represented learning outcomes.
This approach was also utilized to test differences across all 110 neural region parcellations to predict learning outcomes across age groups.
Results (analyzed and visualized in R):
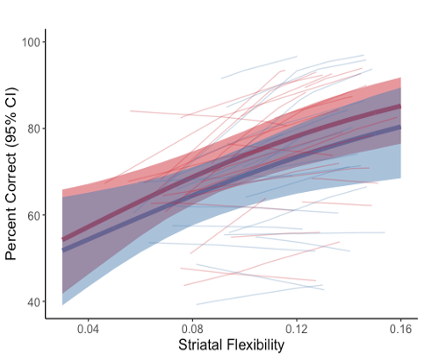
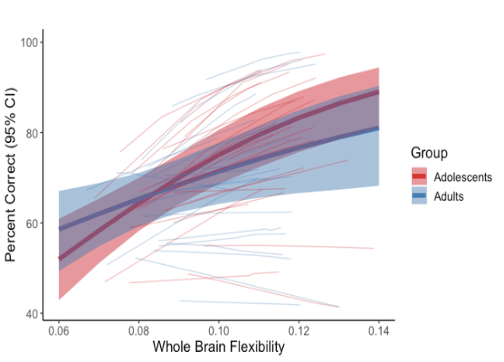
Striatal and average whole brain DNF predicts learning in both age groups. DNF of the striatum predicted learning (β = 12.09, SD = 2.90, 95% CI [6.53, 18.06]) in both age groups, replicating the previously published findings in the adult only data (Gerraty et al., 2018) in the adolescent sample, with no significant interaction between DNF and age group. Average whole brain DNF predicted learning (β = 19.58, SD = 3.82, 95% CI [12.42, 27.56]) across all participants. Bold lines represent model prediction of age group, shaded area represents 95% credibility intervals, and thin lines represent individual subjects’ predictions for each of the 4 blocks.
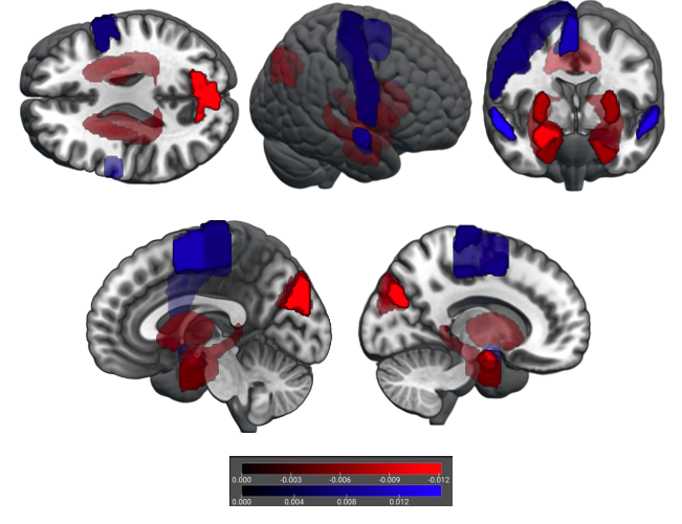
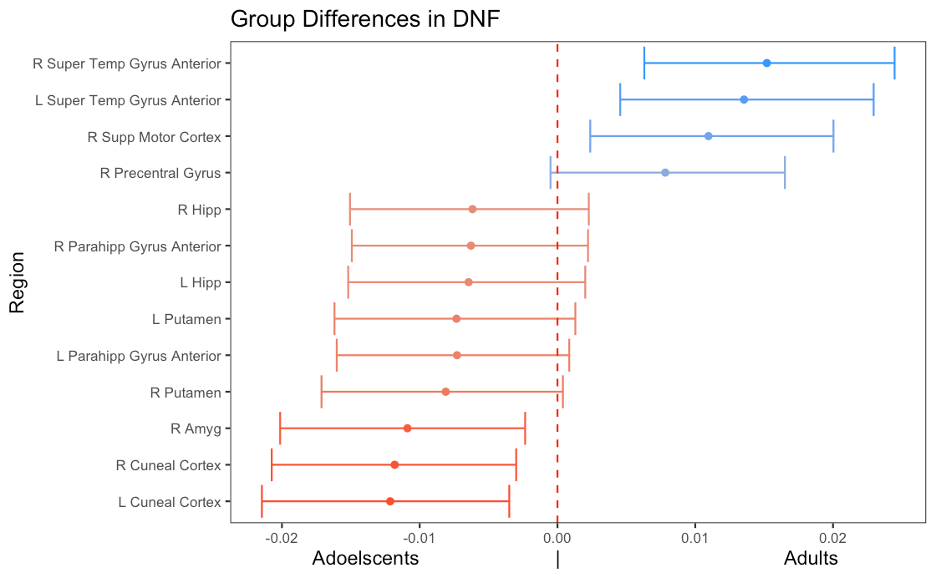
Brain-wide search for age group differences in DNF. A. ROIs with age group differences in DNF displayed on a brain template. Red depicts adolescent > adult DNF and blue depicts adult > adolescent DNF. Abbreviations: L = Left, R = Right, Super = Superior, Temp = Temporal, Supp = Supplementary, Hipp = Hippocampus, Parahipp = Parahippocampal, Amyg = Amygdala. B. ROIs with age group differences in DNF (not related to learning). There were no age group differences in DNF of any ROI predicting learning. However, several ROIs showed age group differences in DNF (in a model that reduced multiple comparison errors by shrinking estimates to the mean) irrespective of learning. Plotted are 95% CI at an 87% CI threshold to emphasize ROIs that were close but did not pass the 90% CI threshold.
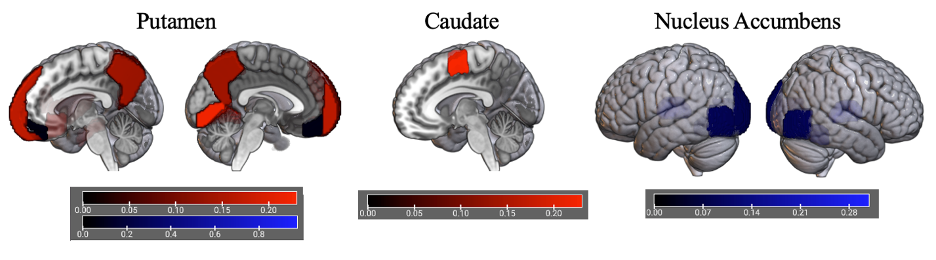
Display of striatal allegiance for both age groups. Red colored ROIs represent the average adolescent group striatal allegiance and blue colored ROIs represent the average adult group striatal allegiance. See Supplementary Figure 4.3 for details on adolescent-only ROIs and see Gerraty et al., (2018)for more details on adult-only ROIs.
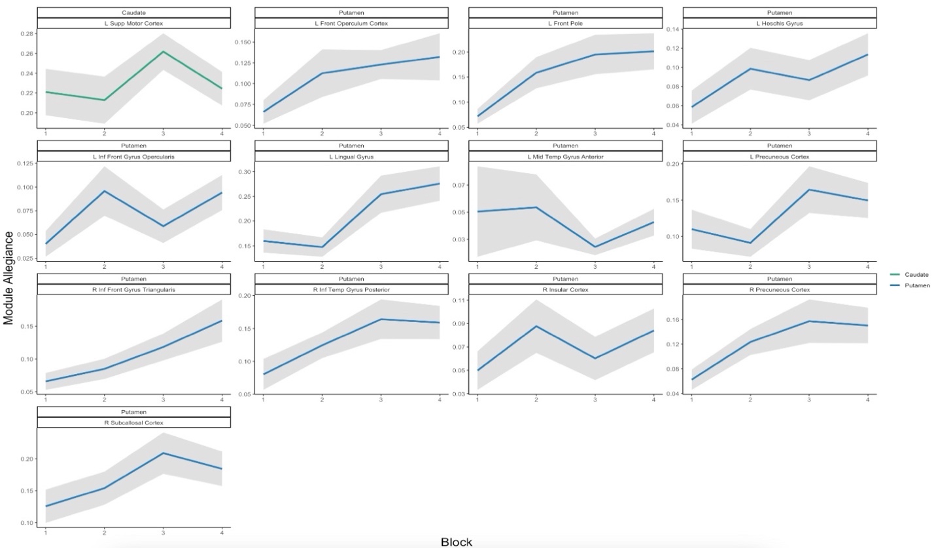
Adolescent striatal ROI allegiance. As was analyzed in adults (see Gerraty et al., 2018), to determine which regions changed coupling with the striatum during the course of the task, we fit mixed-effects ANOVA models using learning blocks to predict log-transformed module allegiance. Module allegiance is the proportion of time windows in which a pair of regions are most strongly coupled with each other at a given point in time, to obtain stable estimates, as was done with flexibility statistics; allegiance scores for each ROI were averaged over the 500 iterations of the multilayer community detection algorithm. Mixed-effects models were performed for each region of the brain, treating blocks as a factor so as to avoid assumptions about the linearity or direction of changes in allegiance, controlling for false discovery rate across all ROI-striatum pairs (pFDR < .05). Analyses were conducted separately for each age group and differing significant ROI-striatum allegiance relationships emerged. Panel plots show regions that demonstrate changes in allegiance over time for every pair passing the correction threshold. Bands represent standard errors. In adults, the nucleus accumbens and the putamen increase coupling with visual regions during the task, in addition the putamen exhibits an increase in coupling with primary auditory cortex, and no regions’ allegiance with the caudate survived correction for multiple comparisons (Gerraty et al., 2018). In adolescents, the caudate increased coupling with the motor cortex across the task, and the putamen increases coupling with various cortical regions. Abbreviations: L = Left, R = Right, Occ = Occipital, Temp = Temporal, Front = Frontal, Inf = Inferior, and Mid = Medial. Abbreviations: L = Left, R = Right, Super = Superior, Temp = Temporal, Supp = Supplementary, Hipp = Hippocampus, Parahipp = Parahippocampal, Amyg = Amygdala, Occ = Occipital, Front = Frontal, Inf = Inferior, and Mid = Medial.
Comments